Landing a spacecraft on the moon can be a dangerous endeavor. The pilot will need to work well with the spacecraft’s automated systems to land safely and on target. Dr. Kevin Duda’s project will measure the effects of both human and automation errors as they spread through a supervisory control system. The researchers will also use models to predict the effects of information displays on pilot-vehicle performance. The end result of the project will be a model-simulation design tool that can be used to identify appropriate task allocation between human and automated control and information requirements needed for safe and successful lunar landings.
Overview
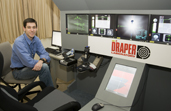
Dr. Kevin Duda sits with fixed-base lunar landing simulator at Draper Laboratory. The simulator is currently being used for Dr. Duda???s NSBRI project investigating human-automation task allocation and the NSBRI Sensorimotor Adaption Team project of Dr. Laurence Young investigating control modes and displays. The simulator provides a research platform to investigate pilot performance during automatic and manually-controlled landings, with primary flight and situation awareness displays as well as out-the-window views with realistic lunar south pole terrain and varying lighting conditions. Image courtesy of Kevin R. Duda, Ph.D. Click here for larger image.
Human-Automation Interactions and Performance Analysis of Lunar Lander Supervisory Control
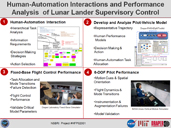
The project is developing an early-stage simulation-based design tool for human-automation interactions in complex systems and validating both fixed- and moving-base lunar landing simulators. This work is a collaborative effort between Draper Laboratory, the MIT Man-Vehicle Laboratory, and NASA Ames Research Center. Image courtesy of Kevin R. Duda, Ph.D. Click here for larger image.
Principal Investigator:
Kevin R. Duda, Ph.D.
Organization:
The Charles Stark Draper Laboratory, Inc.
Technical Summary
The project objective is to produce an integrated human-system model that includes representations of perception, decision making and action for use as an early-stage simulation-based design tool. To support this, we will quantify the effects of both human and automation errors as they propagate through a supervisory control system. We will also look at the effects of functional allocation and information display on mission and pilot-vehicle system performance through dynamic modeling and experimentation.
Specific Aims
- Perform a critical analysis of human operator-automation interactions and task allocations, considering information requirements, decision making and the selection of action.
- Develop a closed-loop pilot-vehicle model, integrating vehicle dynamics, human perception, decision making and action, and analyze using reliability analysis techniques in MATLAB/Simulink to quantify system performance.
- Conduct experiments in the Draper Laboratory fixed-base lunar landing cockpit simulator to validate critical parameters within the integrated pilot-vehicle model.
- Extend the dynamic model to include the effect of spatial orientation on system performance and conduct experiments on the NASA Ames Vertical Motion Simulator to investigate the effects of motion cues on pilot performance.
Key Findings
In project year 2, we completed our initial human-system model development. Our simulation varied the human performance model parameters, and recorded system performance to determine the sensitivity to variations in human activity. Landing accuracy and fuel usage were two system-level parameters to score performance against. Two key findings include: The best combined fuel and landing accuracy was found when the human does the decision making, but aided by automated flight, and variability in fuel savings when the human is flying is greater than automated flying, but the average is not always greater. This supports the human-system collaboration hypothesis that the combined human-automation performance is better than either the human or the automation alone.
We also completed an experiment investigating "graceful" mode transitions within complex systems. Subjects flew trajectories that transitioned from a fully automatic flight control mode to one of three manual flight control modes.
Workload was measured using the Modified Bedford Scale and secondary task response time. Verbal callouts of altitude, fuel, and location – provided a measure of situation awareness (SA). Flight performance was evaluated using the pitch axis tracking error. The key findings include: 1) secondary task response time and subjective workload all significantly increased following the mode transition, 2) the subjects' Modified Bedford reports, when ranked, showed unanimous agreement that workload was lowest prior to the transition, and highest during it, and 3) SA callout accuracy decreased significantly after the transition.
With the new SA metric, we were able to demonstrate for the first time a short term decrease in SA during difficult mode transitions. We also found that workload depended on the number of control loops the subject was required to close.
The results of the human-automation task allocation modeling and simulation supports the human-system collaboration hypothesis that the combined human-automation performance is better than either the human or the automation alone. This work leverages the hierarchical task analysis performed in Aim 1, and expands on Aim 2. In addition to the implementation of human performance models, we developed analogous automation performance models – allowing us to simulate performance under varying task allocations. The results of the simulation informed the landing point designation and human decision making hypotheses for Aim 3 experimentation. Several of these tasks models will be implemented as "typical spacecraft command and control tasks."
The objective of research Aim 1 was expanded to investigate "graceful" transitions between automation modes. Accidents and incidents in aviation, maritime, and space were analyzed to produce a generalized set of design guidelines and metrics to quantify the workload, situation awareness, and system performance changes during mode changes. An experiment in the context of piloted lunar landing was performed to quantify the "gracefulness" of transitions between automation modes. This investigation filled a void in the task allocation analysis literature by considering dynamic task allocation, as well as utilizing a novel method for assessing situation awareness in near-real-time using verbal callout accuracy. The results of this experimentation contribute to Aims 1 and 3, and the system performance, workload, and situation awareness metrics will be used to inform the modeling effort in Aim 2.
Proposed Research Plan
Proposed research plan for the coming year In project year 3, there are four elements of our research plan that we plan to advance:
1) Expand on the model development in Aim 2 to include human failure detection and response to off-nominal scenarios. This will allow us to analyze the system response to degraded states, and analyze the human-automation task allocation and system performance using Draper Laboratory's performance and reliability analysis techniques.
2) Complete the human decision making and action experiment during landing point designation and approach-to-landing tasks. This experiment was started in year 2, and the results will be used to validate the Aim 2 models.
3) Initiate the packaging of the human performance models within MATLAB/Simulink to be a stand-alone library.
4) Coordinate the Aim 4 research plan using the NASA Ames VMS. In addition, we will continue to work with other NSBRI HFP Team investigators to identify potential future collaborations that leverage the Team's expertise and integrate this model-based human-system design work.
Earth Applications
The integrated human-system modeling and human-automation interaction analyses developed by this project are generally applicable to any complex system, whether it is land, air, sea or space-based. The development of the task network and human performance model library in the MATLAB/Simulink environment is an important contribution to the early-stage model-based design approach that utilizes Simulink to represent the system dynamics and capabilities. The formulation of the human as a component in the system under development is critical for the analysis and design of the role of the human in complex systems, where there are interactions with the automated systems and control modes, and while performing critical functions at various levels of supervisory control.
This research project will produce both abstract representations of human performance models to formulate the human as a system component as well as analytic approaches to determine the effect of human and/or automation errors as they propagate through the system and affect mission performance and reliability. Our analyses of automation mode transitions go beyond the space-rated vehicles and includes aviation and nautical accidents/incidents. Documenting and learning from the interactions between the human and the automation will help us develop a generic set of guidelines for the design of system modes as well as produce metrics for quantitatively evaluating the ease and safety of transitioning between modes in both nominal and off-nominal scenarios. This modeling and analysis work can be applied to multiple supervisory control applications such as aircraft, helicopters and unmanned aerial vehicle interactions or explaining the causes of accidents. It may also suggest new methods to assess pilot performance and determine training curriculums.
The research has also developed a new situation awareness metric – one that allows for continuous measurement without interrupting the reporter or simulation. This non-invasive method requires the participant to verbally callout specific vehicle/system states that are pertinent to the task that they are executing, and we record the correctness of the callout. Within a trial this gives a indication of which points they missed callouts, and across trials it provides temporal comparisons of task sequences/events that result in lower situation awareness. This method could be applied to many land-, sea- or space-based systems where there is a need to assess operator situation awareness over time. Specific examples for space operations and exploration may include teleoperation/remote manipulator operation and near-Earth object/asteroid rendezvous and proximity operations.